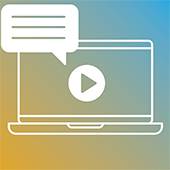
October 3, 2024
Title: Can GenAI for Literature Reviews Ever Be Trusted? A Pragmatic Approach That’s Ethically Designed and Purpose-built for HEOR Professionals
Thursday, October 3, 2024
10:00am EDT | 2:00pm UTC | 4:00pm CEST
Click here for time zone conversion
Description
As healthcare systems become increasingly data-driven, AI is emerging as a critical technology to improve efficiencies and lower costs. But can off-the-shelf GenAI confidently enable HEOR practitioners to deliver trusted results? This webinar will highlight practical use cases and best practices methods in applying Generative and Composite AI methods to meet the demands of health economics and outcomes research (HEOR). Mark Priatel, Chief Technology Officer, and Rushdi Shams, Senior Machine Learning Engineer at DistillerSR will share best practices for the application of Generative and Composite AI methods to literature reviews.
Learning Objectives:
- Learn how FAIR principles can be applied to the design of Composite AI approaches to literature reviews and evidence extraction.
- Assess the pros and cons of off-the-shelf vs. Composite AI offerings in terms of accuracy, traceability, and auditability, and performance.
- Gain insights into how integrating AI within your organization streamlines workflows for faster, more accurate research and decision-making.
Moderator:
Mark Priatel, PhD, Chief Technology Officer, DistillerSR, Canada
Speakers:
Remon van den Broek, MSc, PhD, Head of AI Strategy and Data Science at Adelphi Group, Amsterdam, the Netherlands
Rushdi Shams, PhD, Senior Machine Learning Engineer, DistillerSR, Canada
Brought to you by: DistillerSR
Please note: On the day of the scheduled webinar, the first 1000 registered participants will be accepted into the webinar. For those who are unable to attend, or would like to review the webinar at a later date, the full-length webinar recording will be made available at the ISPOR Educational Webinar Series webpage approximately 2 days after the scheduled Webinar.
Reservations are on a first-come, first-served basis.