Machine Learning Methods Session Draw Large Crowds at ISPOR 2022
Vasco Miguel Pontinha, MPharm, MA, Virginia Commonwealth University School of Pharmacy, Richmond, VA, USA
William Padula, PhD (University of Southern California, USA) opened one of the most exciting sessions of the day titled, “How to Apply Machine Learning in HEOR.” The level of interest in this topic was clearly shown by the large attendance, which went beyond the capacity of the room. The goal of the session was to provide updates about the forthcoming guidance about how to implement machine learning methods in HEOR from ISPOR’s Machine Learning Task Force. This working group was established prepandemic in 2019 and has been working since on how to integrate these methods in HEOR.
The beginning of the session was punctuated by a description of what machine learning is and what it is used for. In short, machine learning methods belong to a large family of methods that are used for classification and prediction purposes.
Tasked with establishing guidance on the implementation of machine learning methods in HEOR, the Task Force also focused its work on identifying critical examples that can be used as case-studies in the HEOR field. The Task Force, Padula explained, focused its work on 6 fundamental aspects: (1) cohort selection; (2) feature selection; (3) predictive analytics; (4) causal inference; (5) economic evaluation; and (6) transparency and explainability.
"While machine learning methods still require human input, the implementation of these methods reduces human abstract time by approximately 57%."
The first aspect, “cohort selection,” was discussed by Blythe Adamson, MPH, PhD (Flatiron Health, USA), who presented a case study about how difficult it is to identify patients for a potential metastatic breast cancer study. If investigators are willing to overcome the limitations of the method of identifying patients by diagnosis codes, machine learning algorithms such as deep learning can be used to scour the medical records. Metastatic breast cancer is a prime example of how difficult patient enrollment is because of the low prevalence and lack of specificity of diagnosis codes. So, the question becomes how likely are researchers able to identify the relevant patient in a cohort? In summary, the deployment of machine learning algorithms, used in combination with human abstraction of medical records, are useful to review medical notes and identify those relevant patients by processing language and specific terms written by the physicians. While machine learning methods still require human input, the implementation of these methods reduces human abstract time by approximately 57%.
Another aspect that Adamson discussed was the "feature selection” component. Since datasets are now highly dimensional and comprise so many individuals, making sure to include only the relevant features that are somewhat related with the outcome or, more importantly, predictive of that outcome becomes a significant challenge. Machine learning methods are able to assist in that endeavor, since some algorithms like random forests and boosted versions establish scores of the level of importance of each covariate for a given outcome.
Don’t try this at home [or at least, not alone!]
Due to the complexity of the challenge, Adamson and Padula suggested that the application of these methods should be done in collaboration with a third party, whether it is a consulting company, software engineers, biostatisticians, or computer scientists.
David Vanness, PhD (University of Pennsylvania, USA) provided some background on how machine learning can be helpful in projects associated with predictive analytics and causal inference. Vanness noted, “As we expand the algorithms to examine the relationships between variables, we need to expand the set of tools that are available.” However, the relationship between variables in order to establish a predictive pattern is tied to a decision that needs to be made. Since a future decision can also be based on existing data that showed that relationship, researchers need to focus on the how much loss (error) of the prediction can incur if the outcome is not correctly predicted. In other words, what is the willingness to accept under- or overestimation of the outcomes? According to Vanness, one of the fundamental aspects that can help reduce this “error” is by only including the elements that actually predict or support that decision—much like Adamson had argued before. This phenomenon (known as the bias-variance trade-off) compares the level of complexity of the model (ie, the number of features) versus the expected error in prediction. Graphically, this relationship is traditionally represented by a U-shape curve. Vanness also discussed how cross-validation techniques, parameter running, and random feature selection can provide useful in improving the predictability of a machine learning-based model.
"What is the willingness to accept under- or overestimation of the outcomes?"
This exciting session was followed by a second intervention by Padula, who talked about the relevance of machine learning methods in HEOR as one way to improve economic modeling. Economic models have specific areas of uncertainty, like structural uncertainty. While receiving input from existing guidelines or clinician feedback can prove useful, Padula challenged the audience by asking the percentage of patients who actually receive guideline-based treatments? By providing an example from the pressure injury field, Padula explained how big data and application of machine learning can be useful in ascertaining how patients are actually treated and from there, identify the relevant predictors for specific outcomes and reduce the number of assumptions in models. Finally, Padula discussed the importance of “transparency and explainability” from researchers implementing machine learning-algorithms. In order to overcome the “black box” perception, researchers should continue working on explaining these newer methods and its results to decision makers and help justify why (particularly unsupervised) methods are appropriate to answer clinical questions.
The panelists closed the session by presenting the general principles of the forthcoming guidance on machine learning in HEOR, which will be published in the July issue of Value in Health. The key aspects of the guidance are shown in the Figure below.
Figure. The PALISADE checklist.
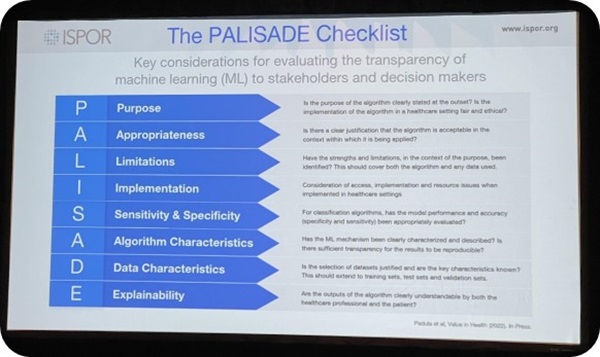