Felicitas Kühne, MSc
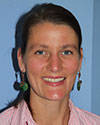
Senior Scientist, Institute of Public Health
Medical Decision Making and Health Technology Assessment Department of Public Health, Health Services Research and Health Technology Assessment, UMIT, Hall i.T., Austria
Since 2011, Felicitas Kühne is as a Senior Scientist in decision-analytic modeling and epidemiology at the Department of Public Health, Health Services Research and Health Technology Assessment at UMIT. She conducts decision-analytic and real-world evidence epidemiologic studies in several disease areas, and teaches courses in advanced epidemiologic methods. She is the Coordinator of the Program on Causal Inference in Science at the Department, and is the Coordinator of the course “Causal Inference in Observational Studies and Clinical Trials Affected by Treatment Switching: A Practical Hands-on Workshop” in the Continuing Education Program on Health Technology Assessment and Decision Science (HTADS) at UMIT. She is currently working on her doctoral thesis in decision science and causal inference. Felicitas completed her Master of Science in Health Policy and Management at the Harvard School of Public Health in 2001. From 2001 to 2011 she worked as a consultant for pharmaceutical companies, conducting several cost-effectiveness analyses in a variety of disease areas. Her research interests include decision analytic modeling, causal inference methods, outcomes research, health technology assessment, and personalized medicine. The main disease areas are cardiovascular diseases, cancer, and infectious diseases including HIV/AIDS and hepatitis C. Her studies at Harvard University were partly sponsored by complementary scholarships of the Carl Duisberg Gesellschaft (1 year) and the Boehringer Ingelheim Stiftung (2 years). In 2013, Felicitas received with her team the third German research award for general medicine of the Dr. Lothar Beyer-foundation with a shared decision making project in the cardiovascular disease area. Felicitas Kühne was awarded a Tyrolean Science Fund (TWF) grant in 2016 for a two-year causal inference project.